Mastering qualitative analysis
Explore the power of qualitative analysis to uncover deep insights and drive strategic decisions in business, education, and social research.
- Qualitative Data Analytics - Power of open-ended data and analysis for program evaluation" by SoPact:
- Importance of Qualitative Data: The video emphasizes that qualitative data is often underused or misunderstood, but it is crucial for gaining deep insights.
- Traditional vs. Modern Data Collection: Traditional methods of collecting quantitative data are complex and time-intensive, often handled by external evaluators, which can distance organizations from their data.
- Three-Tiered Data Collection Process: The innovative approach combines closed-ended questions, open-ended responses, and data segmentation to provide comprehensive insights.
- Top-Down and Bottom-Up Analysis: The methodology integrates inductive (bottom-up) and deductive (top-down) reasoning for a well-rounded understanding of the data.
- Automated Data Collection: Automation and standardization in data collection enhance speed, accuracy, and ongoing learning.
- Breadth and Depth in Data Collection: Effective data collection involves capturing diverse perspectives (breadth) and delving deeply into stakeholders' experiences (depth) to reduce bias.
- Impact Dimensions: Key demographic or categorical factors (e.g., age, location, education) are used to contextualize the data and enable targeted interventions.
- Modern Feedback Analytics: Advanced tools and AI-driven analytics streamline the process of collecting, analyzing, and deriving actionable insights from qualitative data.
- Efficiency and Actionable Insights: Modern feedback analytics connect surveys seamlessly with data analysis, providing summarized qualitative and quantitative data quickly and accurately.
- Crafting Compelling Narratives: The power of modern feedback analytics lies in its ability to transform data into compelling stories that drive change and make a difference.
Qualitative Analysis: An In-Depth Exploration
Qualitative analysis is a research method used in the social sciences and humanities. Qualitative researcher studies people's beliefs, experiences, attitudes, behaviors, and interactions.
Unlike quantitative examining, which focuses on numerical data and statistical interpretation, qualitative understanding seeks to comprehend complex phenomena through non-numerical data. This data can include texts from interviews, observations, photographs, and videos, providing rich, detailed insights into the subject matter.
Data Analysis for Qualitative Data
Qualitative data analysis is a systematic approach to interpreting non-numeric data such as text, audio, and video, aiming to understand the underlying meanings, patterns, and themes. This process involves several key steps to ensure that the analysis is thorough, accurate, and insightful. Here's a breakdown of the essential components of qualitative data analysis:
- Data Familiarization: This initial step involves immersing oneself in the data to gain a comprehensive understanding. It includes transcribing interviews, reading through field notes, and listening to audio recordings multiple times. The goal is to become intimately familiar with the data, noting any initial observations and potential themes.
- Coding: Coding is the process of labeling segments of the data with descriptive tags. These codes can be either predefined (deductive) or emerge from the data itself (inductive). Coding helps in organizing the data into manageable chunks and lays the groundwork for identifying patterns and themes. Tools like NVivo or MAXQDA can be utilized to facilitate this process.
- Identifying Themes: After coding, the next step is to identify overarching themes that capture the essence of the data. This involves grouping similar codes together and looking for patterns or recurring ideas. Themes should be coherent, significant, and supported by the data.
- Interpreting and Analyzing Themes: Once the themes are identified, they need to be interpreted and analyzed in the context of the research question. This involves linking themes to theoretical concepts, understanding their implications, and exploring relationships between different themes. The aim is to construct a narrative that provides a deeper understanding of the data.
- Reporting Findings: The final step is to present the findings in a clear and structured manner. This includes describing the themes, providing evidence from the data to support them, and discussing their relevance to the research question. Visual tools such as concept maps or thematic charts can be used to illustrate the findings effectively.
Methodologies in Qualitative Data Analysis
- Grounded Theory: This inductive approach involves generating theories based on the data itself. Researchers collect and analyze data simultaneously, continuously refining their theories as new data is gathered.
- Phenomenology: This method focuses on understanding individuals' lived experiences and the essence of these experiences. It's particularly useful for exploring deeply personal or subjective phenomena.
- Narrative Analysis: This technique examines the stories or accounts people provide, analyzing how they construct and communicate their experiences. It emphasizes the context and structure of the narratives.
- Content Analysis: This method involves systematically categorizing textual data to identify patterns and themes. It can be both qualitative and quantitative, depending on the research objectives.
Qualitative Data Analysis
Qualitative Data Analysis (QDA) is the systematic process of organizing, interpreting, and making sense of the collected data. This process involves several key steps, each crucial for uncovering patterns, themes, and insights within the data.
QDA involves studying data by describing it in detail rather than using numbers and statistical models as in quantitative analysis. Researchers use qualitative methods to analyze data in QDA.
This approach focuses on understanding the context and meaning of the data. It allows researchers to gain insights and identify patterns that quantitative methods may not capture. Researchers who use QDA examine the data closely and delve into the specifics of the information.
This method helps achieve a more in-depth understanding of the studied data. By focusing on detailed descriptions, researchers can gain insights that quantitative analysis alone may not capture. This deep engagement with the data helps to reveal the underlying meanings and relationships that define the studied phenomena.
Key Steps in Qualitative Data Analysis
- Data Collection: Collecting non-numeric or qualitative research data using customer interviews, focus groups, customer surveys, narrative analysis, and customer feedback observations. T
- Data Organization: Transcribing interviews, organizing field notes, and categorizing visual data for easier analysis.
- Coding: Identifying and labeling themes and patterns within the data.
- Thematic Analysis: Grouping codes into broader themes that capture significant aspects of the data.
- Interpretation: Analyze the themes to understand their implications and the deeper meanings they convey.
- Verification: Ensuring the accuracy and credibility of the findings through methods like triangulation and member checking.
- Presentation: Communicating the findings clearly and compellingly, often through detailed narratives and visual representations.
Benefits of Qualitative Analysis
Qualitative analysis offers several advantages, including:
- Depth of Understanding: Provides a comprehensive understanding of complex phenomena by exploring the context and meaning behind data.
- Flexibility: Adaptable to various research contexts and questions, allowing researchers to modify their approach as new insights emerge.
- Rich Data: Yields detailed, nuanced data that can provide a deeper insight into the subject matter.
- Human Perspective: Captures the human elements of experience, often overlooked in quantitative research.
Qualitative data analysis helps researchers better understand social life, making it a crucial tool in the social sciences and humanities. Researchers can uncover the rich, complex, and often surprising realities of human behavior and interactions through careful and systematic qualitative data examination.
Qualitative Data Analysis Methods
Qualitative data analysis methods are essential for making sense of non-numerical data, such as text, audio, and video. These methods help in identifying patterns, themes, and insights from qualitative data to inform decision-making and improve program outcomes. Here are some key qualitative data analysis methods:
- Content Analysis: This method involves systematically coding and categorizing textual data to identify patterns, themes, or biases. Content analysis is useful for analyzing written communications like interviews, open-ended survey responses, or documents.
- Thematic Analysis: Thematic analysis focuses on identifying and analyzing themes within qualitative data. Researchers code the data and group codes into themes that capture important aspects of the data related to the research questions.
- Narrative Analysis: This method examines the stories people tell to understand how they make sense of their experiences. Narrative analysis is particularly useful in fields like psychology, anthropology, and sociology.
- Grounded Theory: Grounded theory is an inductive approach that involves generating theories based on the data collected. Researchers collect and analyze data simultaneously, allowing emerging theories to guide subsequent data collection.
- Phenomenological Analysis: This method aims to understand the lived experiences of individuals from their perspectives. Phenomenological analysis involves in-depth interviews and seeks to describe the essence of these experiences.
Qualitative Analysis Techniques
Qualitative analysis techniques provide tools and strategies to process and interpret qualitative data effectively. Here are some commonly used qualitative analysis techniques:
- Coding: Coding is the process of labeling and organizing qualitative data to identify different themes and patterns. Codes can be predefined or developed inductively based on the data.
- Memo Writing: Researchers use memo writing to capture their thoughts, insights, and reflections during the data analysis process. Memos help in developing a deeper understanding of the data and the relationships between codes and themes.
- Data Visualization: Visual techniques like mind maps, flow charts, and concept maps can help in organizing and presenting qualitative data. These visualizations aid in identifying connections and patterns within the data.
- Constant Comparative Method: This technique involves comparing different pieces of data to identify similarities and differences. It is often used in grounded theory to refine and develop theoretical concepts.
- Qualitative Software Tools: Software tools like NVivo, Atlas.ti, and MAXQDA assist researchers in managing, coding, and analyzing qualitative data. These tools provide features for data organization, visualization, and collaboration.
Qualitative Data Analysis Steps
While there is no one-size-fits-all approach to qualitative data analysis, researchers can follow some common steps to analyze their data effectively.
Step 1: Organize and Prepare the Data
The first step in qualitative data analysis is to organize and prepare the data for analysis. This means writing down interviews, focus groups, or other data and arranging them to make studying easier. You can collect data using several techniques. One of the most popular approaches is a survey, but it is not limited to it.

Step 2: Familiarize Yourself with the Data
Before diving into the analysis, it is essential to familiarize yourself with the data. This involves reading the data multiple times to understand the overall themes and patterns. It can also be helpful to take notes and jot down initial thoughts and ideas.
Step 3: Coding the Data
Coding is the process of labeling and categorizing data to identify common themes and patterns. There are two types of coding: open coding and axial coding.
- Open coding involves breaking the data into smaller units and assigning codes to each. These codes can be words, phrases, or concepts that capture the essence of the data.
- Axial coding involves organizing the codes into broader categories and identifying relationships between them.

Step 4: Identify Themes and Patterns
Once the data has been coded, the next step is to identify themes and patterns. This involves looking for commonalities and connections between the codes and organizing them into broader themes.
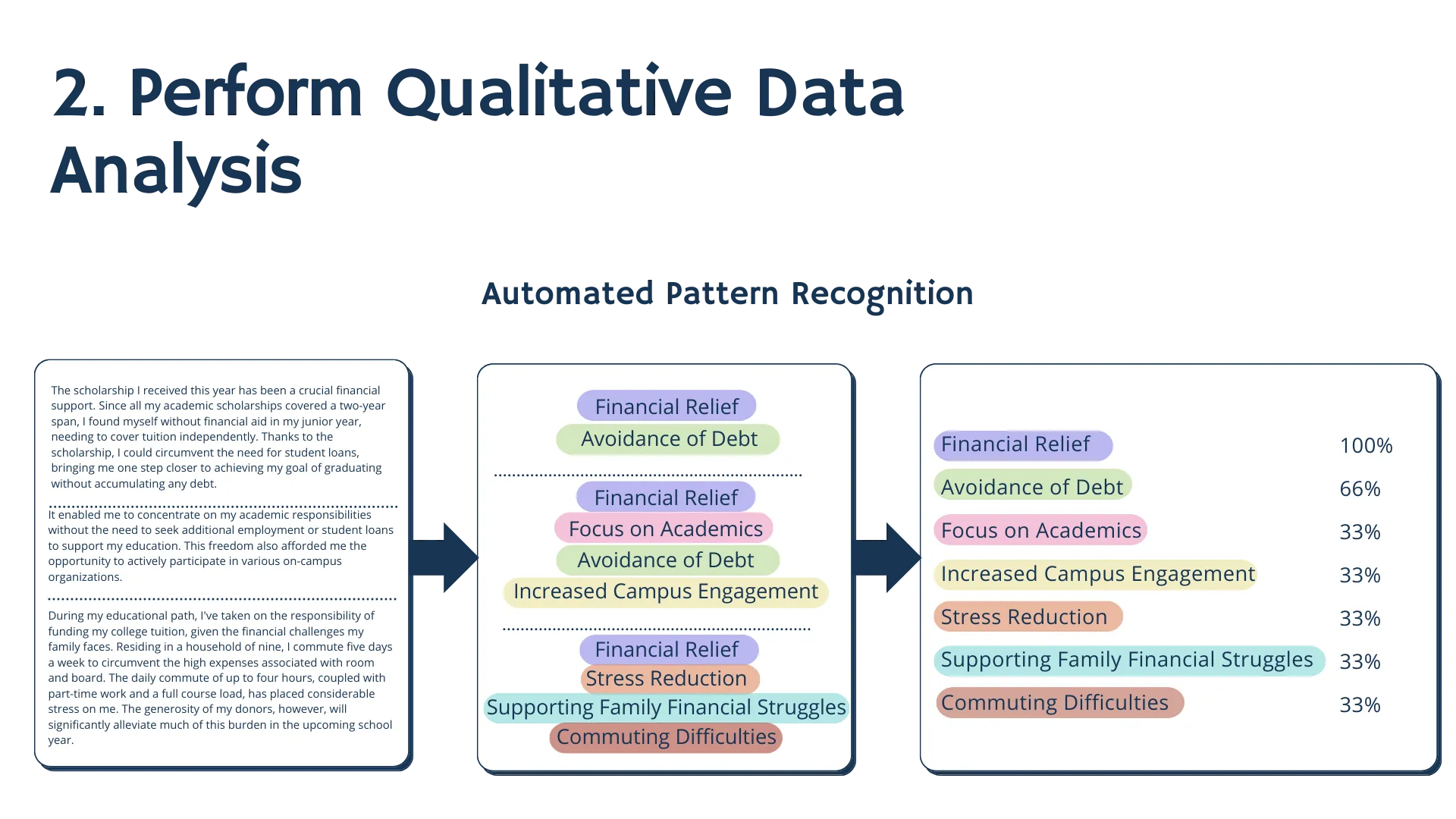
Step 5: Interpret the Data
The final step in qualitative data analysis is to interpret the data. This involves making sense of the themes and patterns identified and drawing conclusions based on the data. It is essential to remain open-minded and consider alternative interpretations of the data.

Qualitative Data Analysis Examples
Qualitative data analysis can be applied in various contexts to derive meaningful insights. Here are some examples of qualitative data analysis:
- Program Evaluation: In a program evaluation for an educational initiative, open-ended survey responses from teachers and students are analyzed to understand their experiences and identify areas for improvement. Thematic analysis reveals common themes such as teaching methods, curriculum effectiveness, and student engagement.
- Healthcare Research: A study exploring patient experiences with chronic illness might use narrative analysis to examine patient stories. This analysis can uncover themes related to coping strategies, patient-provider relationships, and the impact of illness on daily life.
- Marketing Research: In a marketing study, focus group discussions with consumers are analyzed using content analysis to understand perceptions of a new product. Coding the transcripts reveals key themes such as product features, brand perception, and purchasing behavior.
- Social Science Research: A phenomenological study on the experiences of immigrants adapting to a new country might involve in-depth interviews. The analysis focuses on the essence of their experiences, highlighting themes like cultural integration, identity, and social support.
- Policy Analysis: In a policy analysis project, public comments on proposed legislation are analyzed using grounded theory. This method helps in identifying the main concerns, suggestions, and opinions of different stakeholders, providing a foundation for policy recommendations.
The Role of Technology in Qualitative Data Analysis
Advancements in technology, specifically artificial intelligence (AI), are transforming the landscape of qualitatively analyzing data. AI tools can analyze lots of data quickly, saving time for deeper analysis and understanding.
Automated Coding
One of the most time-consuming parts of qualitative data coding is the data. AI tools can automate this process by using NLP algorithms to identify and categorize themes and patterns in the data. This not only saves time but also reduces the risk of human error.
Sentiment Analysis
Sentiment analysis is another area where AI is making an impact in qualitative data analysis. This involves using NLP algorithms to analyze the tone and emotion behind words and phrases in the data. This can provide valuable insights into how participants feel about a particular topic or experience.
Data Visualization
Data visualization tools like charts and graphs can help researchers better understand and communicate their findings. AI-powered tools can automatically generate visualizations based on the data, making it easier to identify patterns and trends.
Real-World Examples of AI in Qualitative Data Analysis
- IBM Watson: IBM Watson is a cognitive computing platform that uses AI to analyze large volumes of data, including qualitative data. It can identify themes and patterns in the data and provide insights to help researchers make sense of their findings.
- NVivo: NVivo is a qualitative data analysis software that uses AI to automate coding. It can also perform sentiment analysis and generate visualizations to help researchers better understand their data.
- Lexalytics: Lexalytics is a text analytics software that uses AI to analyze unstructured data, such as open-ended survey responses. It can identify themes and sentiments in the data and provide insights to help researchers make sense of their findings.
The Future of Qualitative Data Analysis
AI's role in qualitative data analysis will only grow as technology advances. AI tools will better analyze vast amounts of data, helping researchers find crucial insights more easily.
However, it is important to note that AI should not replace human analyzing entirely. Interpreting and understanding data still require human input and critical thinking. AI can assist in the process, but it cannot replace the unique perspective and insights humans bring.
Conclusion
Analyzing qualitative data is important in research. Technology advancements make it easier and faster to analyze this. Researchers can use AI tools to learn important information from their data and make smart decisions.
The steps outlined in this article can help guide researchers through the process. Researchers can analyze their data and conclude to make better decisions by following these steps.