Qualitative Data Analysis Methods: From Traditional Approaches to AI-Driven Insights
Qualitative data analysis (QDA) has long been a cornerstone of research in fields like social sciences, ESG reporting, and impact measurement. Traditionally, this process involves manually coding and analyzing large volumes of textual data, which can be both time-consuming and prone to inconsistencies. These challenges make it difficult to extract timely insights, especially when dealing with complex and diverse datasets.
Recent advancements in AI-driven text analysis have revolutionized this field by significantly reducing the time required for analysis. AI tools can quickly identify themes, patterns, and insights across large datasets, saving researchers hundreds of hours that would otherwise be spent on manual coding and analysis.
Furthermore, the evolution of survey analytics offers an even more streamlined approach by optimizing data collection. Modern survey tools enable continuous and scalable data gathering, transforming the traditional, often static, QDA process into one that is more dynamic and efficient. This approach not only accelerates the analysis but also ensures that insights remain relevant in rapidly changing environments.
Traditional Qualitative Data Analysis Challenges
Narrative analysis stands as a powerful method in qualitative research, focusing on the stories people tell to illuminate their lived experiences. This approach has proven invaluable in fields such as sociology, psychology, and anthropology, where personal accounts offer rich, contextual data that can reveal deep insights into human experiences and social phenomena.
Traditional Qualitative Data Analysis (QDA) software has long been a cornerstone in facilitating narrative analysis. Tools like NVivo, ATLAS.ti, and MAXQDA provide researchers with robust platforms to organize, code, and analyze narrative data. These software solutions support the entire process, from data collection to the final interpretation of findings.
The journey of narrative analysis typically begins with data collection, often through interviews or written narratives. QDA software assists in this initial stage by providing features for audio recording and secure data storage. Once collected, these narratives are usually transcribed, a process that some QDA tools can assist with through integration with transcription services or built-in transcription features.
Narrative Analysis in Qualitative Research
As researchers delve into the analysis, QDA software becomes instrumental in identifying key themes and plot elements within the narratives. These tools offer various coding mechanisms, allowing researchers to mark and categorize different aspects of the stories. Advanced features like auto-coding can help identify recurring themes across multiple narratives, though human insight remains crucial in refining and interpreting these automated suggestions.
The analysis of narrative structure and linguistic features is another area where traditional QDA software excels. These tools often include features for linguistic analysis, allowing researchers to examine word frequency, sentiment, and language patterns. Visualization tools within the software can help researchers map out narrative structures, making it easier to identify common patterns or unique elements across different stories.
Finally, in the interpretation phase, QDA software aids researchers in synthesizing their findings and exploring the deeper meanings and cultural contexts of the narratives. Features like memo writing, data linking, and theory building tools support researchers in developing and documenting their interpretations. While the software provides the framework and tools for analysis, the critical thinking and interpretative skills of the researcher remain at the heart of deriving meaningful insights from narrative data.

The Evolution of Thematic Content Analysis in the AI Era
Thematic content analysis has long been a cornerstone of qualitative research, offering insights into patterns and themes within diverse data sources such as interview transcripts, field notes, and open-ended survey responses. Traditionally, this process involved meticulous manual coding and analysis, often requiring weeks or months to complete for large datasets.
In recent years, the integration of artificial intelligence and machine learning has revolutionized thematic analysis. AI-driven tools now assist researchers in rapidly processing vast amounts of qualitative data, significantly reducing the time and effort required for initial coding and theme identification. For instance, platforms like Sopact Sense leverage natural language processing to automatically suggest codes and themes, allowing researchers to focus on higher-level analysis and interpretation.
One of the most significant advancements is the seamless integration of these AI tools with common data storage platforms. Many modern thematic analysis tools can directly interface with spreadsheet applications, making it easy to import and analyze data from various sources. This integration streamlines the workflow, allowing researchers to move effortlessly from data collection to analysis within a single ecosystem.
The AI revolution in thematic analysis doesn't replace human insight but rather enhances it. These tools excel at identifying patterns and relationships that might be overlooked in manual analysis, especially in large datasets. However, the critical role of the researcher in interpreting these AI-generated insights, refining themes, and contextualizing findings within broader theoretical frameworks remains paramount.
Perhaps most exciting is the emergence of AI-assisted storytelling in the reporting phase of thematic analysis. Advanced algorithms can now help craft initial narratives based on the identified themes, complete with relevant quotes and data visualizations. This feature accelerates the report-writing process and ensures that key insights are prominently featured. However, researchers still play a crucial role in shaping the final narrative, ensuring that the nuances and complexities of the data are accurately represented.
As we look to the future, the potential for AI in thematic content analysis is boundless. From real-time analysis of social media trends to processing multilingual datasets, these tools are opening new frontiers in qualitative research. While challenges remain, particularly in ensuring the ethical use of AI and maintaining the depth of human interpretation, the integration of AI in thematic analysis represents a significant leap forward in our ability to understand and derive meaning from complex qualitative data.

Qualitative Data Analysis Example
A qualitative data analysis report typically includes:
- Introduction and research questions
- Methodology description
- Presentation of key themes or narratives
- Supporting quotes or excerpts
- Discussion of findings
- Conclusions and implications
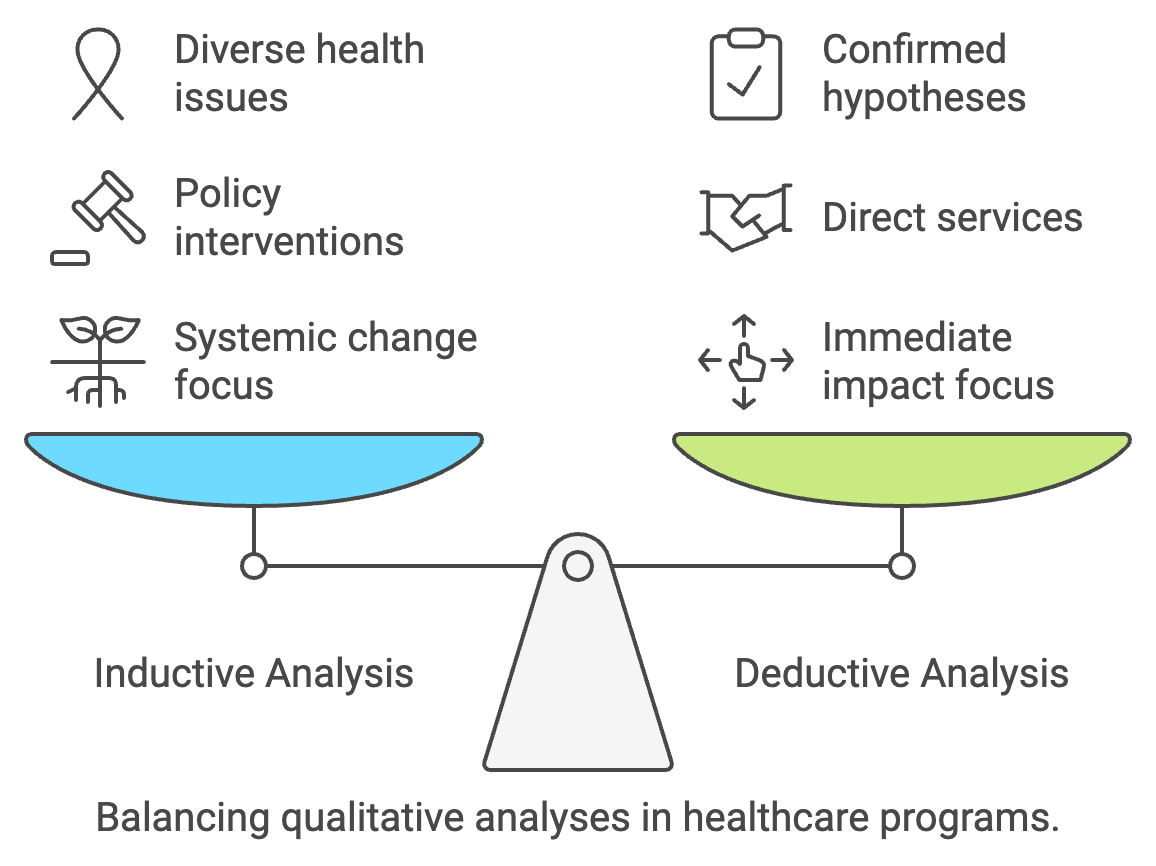
Storytelling: The Impact of Our Grantees
Across urban neighborhoods and rural communities, our 200 grantees are weaving a tapestry of health and hope. From mobile clinics bringing vital care to remote areas to advocates walking the halls of power, each program tells a story of change.
Consider the Healthcare Implementation Program, which has brought mobile health clinics to underserved communities. In one small town, a mother of three finally received the prenatal care she desperately needed, potentially saving her unborn child's life.
Or look at the Mental Health Advocacy Program, which has been instrumental in breaking down stigma. Thanks to their efforts, a young man in crisis found the courage to seek help, turning his life around and becoming an advocate himself.
These stories, multiplied across 200 grantees and countless communities, paint a picture of a healthier, more equitable future. They remind us that behind every data point is a human life changed for the better

Qualitative Data Analysis Challenges
While traditional qualitative analysis methods offer depth and nuance, they come with significant challenges:
- Time-consuming nature of manual coding
- Potential for researcher bias
- Difficulty in analyzing large volumes of data
- Inconsistency in coding across multiple researchers
- Limited scalability for big data projects
The Shift to AI-Driven Qualitative Data Analysis
Textual Data Analysis with AI
AI-driven textual data analysis tools are revolutionizing the field by offering:
- Rapid processing of large volumes of text
- Automated theme identification
- Sentiment analysis
- Pattern recognition across multiple documents
- Reduction of human bias in initial coding
Qualitative Survey Analysis: Transitioning to Structured Data Collection
The landscape of qualitative research is evolving, with a notable shift from purely unstructured data collection methods to a more balanced approach that incorporates structured elements. This transition, particularly in the realm of qualitative surveys, offers researchers a powerful toolset to enhance their data collection and analysis processes. By leveraging a mix of structured and unstructured data through qualitative surveys, researchers can unlock several key advantages that streamline their work while maintaining the depth and richness characteristic of qualitative research.
One of the primary benefits of this transition is the ease of data collection and organization. Traditional qualitative methods often result in vast amounts of unstructured text, which can be time-consuming to process and analyze. By incorporating structured elements into qualitative surveys, researchers can collect data in a more organized format from the outset. This approach not only saves time during the data collection phase but also significantly reduces the effort required to prepare data for analysis.
The inclusion of structured elements in qualitative surveys also allows for more standardized responses to certain questions. While maintaining open-ended questions for rich, detailed responses, researchers can include structured questions that yield easily comparable data across respondents. This standardization facilitates more straightforward analysis and allows for the quantification of some qualitative insights. For instance, Likert scale questions or multiple-choice options can provide quantifiable data alongside more detailed qualitative responses, offering a more comprehensive view of the research subject.
Another significant advantage of this approach is the ability to leverage automated tools for faster initial analysis. While the nuanced interpretation of qualitative data still requires human expertise, the structured elements of modern qualitative surveys can be quickly processed by analytical software. This initial automated analysis can highlight trends, patterns, and key themes, providing researchers with a valuable starting point for deeper, more interpretive analysis. The time saved in this initial processing allows researchers to focus their efforts on the more complex aspects of qualitative interpretation.
Lastly, the transition to a more structured approach in qualitative surveys greatly enhances the ability to compare data across multiple respondents or time periods. With consistent question formats and data structures, researchers can more easily identify trends, changes over time, or differences between groups. This comparative capability is particularly valuable in longitudinal studies or when analyzing responses from diverse populations. For example, a foundation collecting data from multiple grantees can easily track progress and compare outcomes across different programs or time frames, leading to more informed decision-making and strategy development.
In conclusion, the shift towards incorporating structured elements in qualitative surveys represents a significant advancement in qualitative research methodologies. By combining the depth of traditional qualitative approaches with the efficiency of structured data collection, researchers can conduct more comprehensive, efficient, and insightful analyses. This evolution in qualitative survey analysis not only saves time and resources but also enhances the overall quality and applicability of research findings.

Steps in AI-Assisted Qualitative Research
- Data Collection: Gather reports, transcripts, or survey responses.
- Data Preparation: Convert all data to an analyzable text format.
- AI-Based Inductive Coding: Use AI tools to identify initial themes and patterns.
- Human Review and Refinement: Experts review and adjust AI-generated codes.
- Comprehensive Coding: Apply the refined coding framework to all data.
- Aggregation and Analysis: Use AI tools to aggregate findings and identify overarching patterns.
- Narrative Integration: Combine AI insights with human interpretation for rich, contextualized findings.
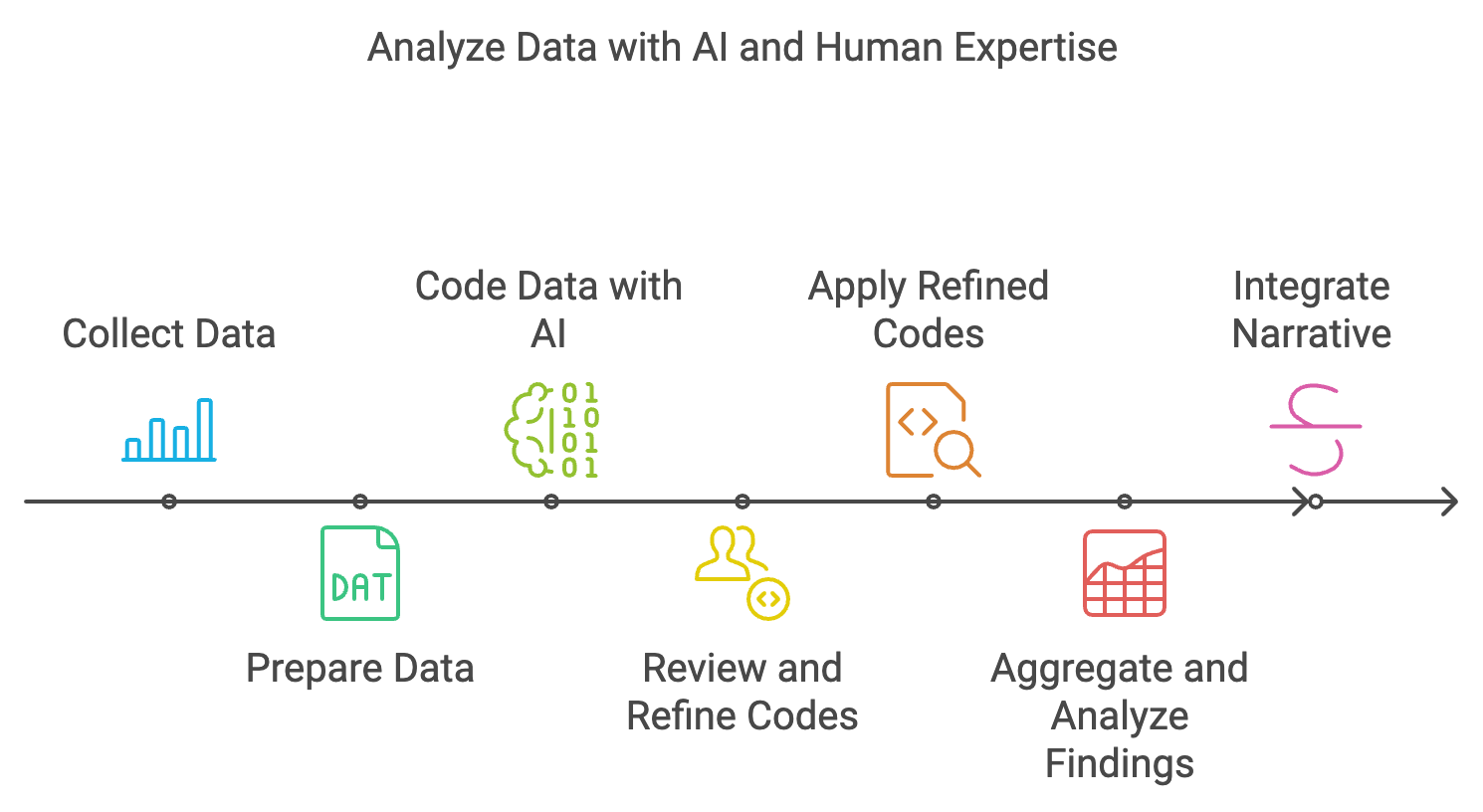
Moving from Interview-Based Analysis to Survey Analytics
Transitioning from traditional transcript-based analysis to survey analytics offers significant efficiency gains and real-time advantages. AI-based text analysis accelerates data processing, but narrative data collection remains challenging. Unstructured data from interviews can be time-consuming to collect and analyze. Below is a comparison between traditional methods and AI-assisted approaches:
Example: Analyzing 200 Grantee Reports for a Foundation's ESG Initiatives
Traditional Method:
- Manual coding of each report.
- Time-consuming theme identification.
- Potential for inconsistency across reports.
AI-Assisted Method:
- Rapid processing of all 200 reports.
- Automated identification of common themes and unique insights.
- Consistent analysis across the entire dataset with human review for context and nuance.
Transitioning to Survey Analytics
The shift from traditional interview-based approaches to survey analytics in ESG reporting offers substantial advantages and some challenges. Understanding both is crucial for optimizing data collection and analysis.
Benefits of Survey Analytics
- Efficiency: Streamlined data collection and analysis reduce time spent on manual transcription and coding.
- Immediate Insights: Real-time data collection and automated analysis enable faster decision-making.
- Scalability: Surveys can reach larger sample sizes more easily than individual interviews, providing broader perspectives.
- Standardization: Structured surveys ensure consistency in data collection, improving reliability in comparisons and trend analysis.
- Cost-Effectiveness: Automated tools reduce the need for extensive human resources in data collection and initial analysis.
Challenges and Considerations
While beneficial, transitioning to survey analytics presents challenges such as:
- Complex Narrative Reporting: Rich, qualitative narratives may lose depth and context when converted to structured data.
- Unstructured Data: Open-ended responses require advanced qualitative analysis tools to capture the full range of themes and sentiments.
- Small-Scale Initiatives: Diverse feedback formats and limited data may not justify the shift to structured analytics.
- Longitudinal Studies: Complex, evolving data may not fit neatly into structured survey formats.
To address these challenges, organizations should consider:
- Hybrid Approaches: Combining traditional qualitative methods with structured surveys where appropriate.
- Advanced Analytics Tools: Investing in sophisticated text analysis tools for better handling of unstructured data.
- Customized Survey Design: Creating tailored, flexible survey instruments to capture nuanced information for complex topics.
- Preserving Narrative Elements: Incorporating open-ended responses within structured surveys to maintain depth of insight.
- Stakeholder Education: Helping stakeholders understand the value of both structured and unstructured data collection methods.
By recognizing these challenges and adapting strategies, organizations can balance the efficiency and scalability of survey analytics with the depth and richness of traditional qualitative methods, tailoring the approach to each reporting context.
Let's take look few examples below to understand which use cases are ideal in-person interviews vs real-time survey based data integration.
The Future of Qualitative Data Analysis
As AI technology continues to advance, we can expect:
- More sophisticated natural language processing capabilities
- Improved integration of qualitative and quantitative data analysis
- Real-time analysis of ongoing data streams
- Enhanced visualization tools for complex qualitative insights
- Greater accessibility of advanced analysis tools for smaller organizations
However, it's crucial to maintain a balance between AI efficiency and human insight. The future of qualitative data analysis lies in the synergy between AI's processing power and the irreplaceable human ability to understand context, emotion, and subtle meanings.
Conclusion
The transformation of qualitative data analysis methods through AI integration represents a significant leap forward in our ability to derive meaningful insights from complex, unstructured data. While traditional methods remain valuable for certain types of in-depth analysis, the incorporation of AI-driven tools offers unprecedented efficiency and scalability, particularly in fields like ESG and social impact research where timely, comprehensive analysis is crucial.
By embracing this evolution in qualitative data analysis, researchers and organizations can unlock deeper insights, identify patterns across larger datasets, and make more informed decisions. As we move forward, the key to success will be finding the right balance between leveraging AI capabilities and preserving the nuanced understanding that human researchers bring to qualitative analysis.