What is Qualitative Measurement?
Ever feel like you're telling the same old story about your program's impact? Numbers are great, but they don't capture the whole picture, do they? What if you could truly understand the "why" behind the "what"?
That's where qualitative measurement comes in. It's about going beyond the numbers and diving deep into the rich tapestry of human experience. Think interviews, focus groups, and observations – all designed to capture the thoughts, feelings, and motivations of the people your programs touch.
But here's the thing: the world is changing fast. Those traditional methods, while valuable, can be slow and cumbersome. They often rely on one-off evaluations, leaving you stuck with a static picture of your program's impact. What if you could get real-time insights and continuously improve your programs?
Enter continuous learning with AI! Imagine using cutting-edge technology to automate data collection and analysis. This isn't just about fancy gadgets – it's about empowering you to:
- Hear stakeholder voices loud and clear: Gain real-time insights into what matters most to your stakeholders, allowing you to adapt and improve your programs on the fly.
- Demonstrate program effectiveness in a whole new way: Move beyond static reports and tell a compelling story about the ongoing impact your program is making.
- Secure stakeholder support with confidence: Show them you're actively listening and continuously working to make a difference.
This article will be your guide to the modern approach to qualitative data collection. We'll break down the limitations of traditional methods and explore how AI can revolutionize how you gather and analyze insights. By the end, you'll be equipped to harness the power of continuous learning and ensure your programs are constantly evolving to meet the needs of your stakeholders.
Ready to ditch the static and embrace continuous improvement? Let's dive in!
Exploring Methodologies in Qualitative Measurement
Unveiling the "Why"
We talked about ditching the static and embracing continuous program improvement through qualitative data collection (cue dramatic music!). But how do you actually uncover those rich human experiences that traditional methods often miss?
That's where different methodologies in qualitative measurement come into play. Each approach offers a unique lens for capturing the "why" behind the "what" of your program's impact. Let's explore some of the most powerful tools in your continuous learning toolbox:
1. Living in Their World: Ethnography
Imagine becoming a fly on the wall, observing a community firsthand. That's the essence of ethnography. This method involves immersing yourself in the context you're studying, participating in activities, and observing behaviors. By living alongside your stakeholders, you can gain a deeper understanding of their culture, values, and experiences with your program.
2. The Power of Storytelling: Narrative Analysis
People love stories, and for good reason! Narrative analysis delves into the stories individuals tell about their experiences with your program. By analyzing the content and structure of these narratives, you can uncover hidden themes, motivations, and emotional responses. Think of it as unlocking the deeper meaning behind the words.
3. Walking in Their Shoes: Phenomenological Research
Ever wonder, "What's it like to be in their shoes?" Phenomenological research helps you do just that. Through in-depth interviews, you explore the lived experiences of your stakeholders in relation to your program. This method gets to the heart of their perceptions and how your program has impacted their lives.
4. Building Your Own Theory: Grounded Theory
Let's say you're starting with a hunch about your program's impact, but need more evidence. Grounded theory is your friend! This methodology involves iteratively collecting and analyzing data, allowing a theory to emerge organically from the ground up. You'll be surprised by the rich insights that can surface through this systematic approach.
5. Deep Dives: Case Studies
Need to understand a specific program outcome in detail? Case studies offer a powerful lens. By examining a single case or a few closely related cases, you can gain a comprehensive picture of the context, challenges, and successes of your program in action. This detailed analysis allows you to learn from specific situations and improve your program for future iterations.
Remember, each methodology has its strengths and weaknesses. The best approach often involves combining these techniques to create a well-rounded understanding of your program's impact.

Exploring Methodologies in Qualitative Measurement
and Why We Need a Modern Approach
We talked about ditching the static and embracing continuous program improvement through qualitative data collection. But let's be honest, some traditional methods can feel...well, a bit traditional. While ethnography, narrative analysis, and case studies offer valuable insights, they often come with limitations.
The Challenges of Traditional Methods:
- Third-Party Dependence: These approaches often rely on external researchers, leading to a loss of control and potential for external biases creeping in.
- Time-Consuming Processes: Traditional methods can take months, even years, to complete, hindering your ability to adapt and improve your program quickly.
- Limited Depth and Frequency: In-depth interviews and focus groups, while valuable, are typically infrequent, potentially missing the full picture and failing to capture ongoing changes in stakeholder experiences.
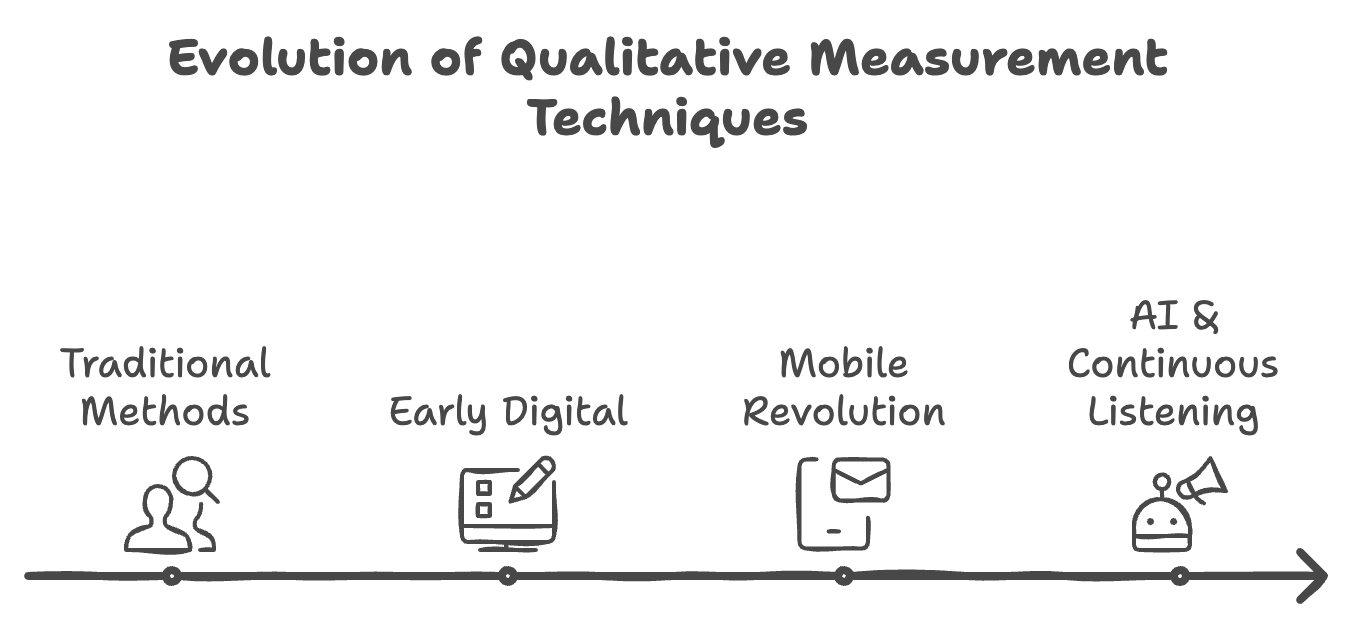
The Future of Qualitative Measurement: Continuous Listening
Thankfully, the field of qualitative data collection is evolving! The future lies in continuous listening to your stakeholders. Imagine:
- Frequent data collection: Gather insights regularly through SMS, WhatsApp, or email, keeping your finger on the pulse of stakeholder experiences.
- Shorter, more focused surveys: Move away from lengthy questionnaires. Use a mix of closed-ended and open-ended questions to capture both specific data and rich stakeholder perspectives.
- Faster data collection cycles: Modern tools allow for quicker data collection and analysis, enabling you to adapt your program based on real-time insights.
- Advanced qualitative data analytics: New technologies unlock deeper understanding by analyzing longitudinal results, combining qualitative and quantitative data, and delivering actionable recommendations.
This future is about gathering frequent, in-the-moment data directly from stakeholders, painting a more complete picture of their experiences and your program's impact.
The methodologies we've discussed are valuable foundations, but the future is continuous. In the next article, we'll explore how to leverage modern tools and techniques to implement continuous listening and unlock the true power of qualitative data collection for your organization.
Qualitative Measurement Examples
Qualitative measurements provide rich, detailed data about experiences, behaviors, and perceptions that can't be easily quantified. These methods allow researchers to explore the depth and nuance of human experiences and social phenomena. The landscape of qualitative research is evolving, blending traditional techniques with cutting-edge AI-driven approaches.
The following visual guide illustrates seven qualitative measurement techniques, ranging from established methods to innovative AI-powered solutions. These examples showcase how qualitative data collection and analysis are being transformed across various fields, including education, healthcare, and social impact programs.
By understanding these methods, researchers and program managers can choose the most appropriate approach for gathering in-depth, contextual data to answer their research questions and drive meaningful improvements in their initiatives. The integration of AI and continuous data collection methods is opening new possibilities for more responsive, data-driven decision-making in social impact measurement.
The Bottom Line: A New Era of Understanding and Impact
AI-driven qualitative measurement isn't just an upgrade; it's a complete transformation. By embracing this revolution, organizations can ensure their strategies and actions are consistently aligned with evolving needs and perspectives, paving the way for more profound and lasting social change.
Ready to unlock the power of AI-driven qualitative data collection? Stay tuned for the next articles in this series, where we'll delve deeper into specific use cases and practical tips for implementation!
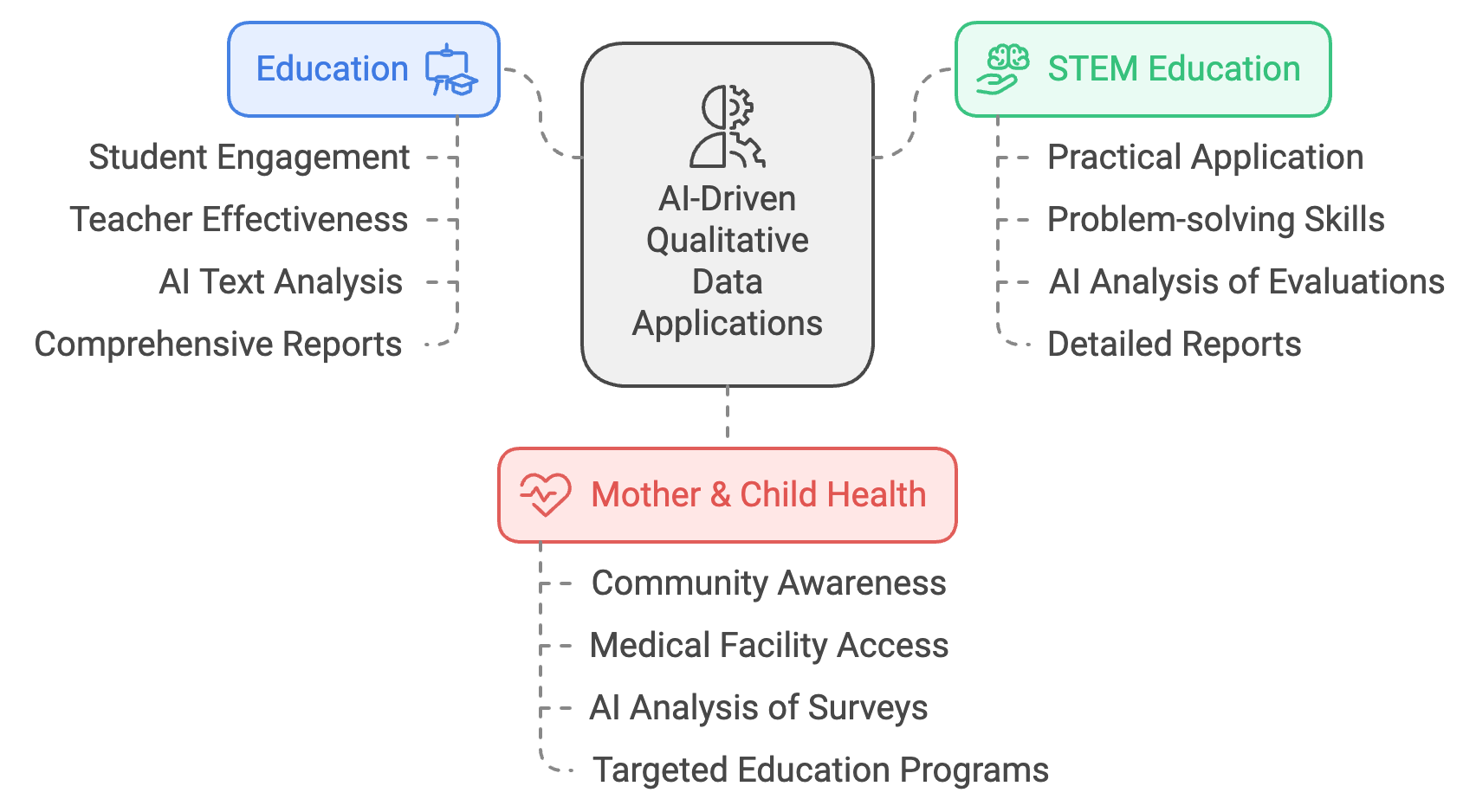
How to Measure Qualitative Data in the Modern Era
Qualitative data goes beyond the numbers, capturing the rich tapestry of human experience. It explores the "why" behind the "what," delving into user attitudes, motivations, and the stories behind the data points [1]. Unlike quantitative data, it's not easily measured with a ruler – but that doesn't mean it's any less valuable.
Modern Approaches to Qualitative Data Collection:
The good news? Gone are the days of solely relying on time-consuming focus groups and interviews. Modern qualitative data collection embraces a variety of methods, including:
- Short, Frequent Surveys: Move away from lengthy questionnaires. Use a mix of closed-ended and open-ended questions delivered via SMS, WhatsApp, or email to gather real-time insights from a wider range of stakeholders.
- Social Listening: Tap into the wealth of data available on social media platforms. Analyze online conversations to understand public sentiment and gain insights into program perception.
- In-App Feedback: Gather feedback directly within your program or website. Offer quick surveys or polls to capture user experiences while they're fresh in mind.
- User Testing: Observe users interacting with your program or product. See firsthand how they navigate the experience and identify areas for improvement.
Extracting Meaning from Your Data: AI-Powered Analysis
Once you've collected your qualitative data, it's time to unlock its potential. Here's where AI comes into play:
- Automated Coding and Analysis: AI tools can quickly categorize and analyze large volumes of text data, identifying recurring themes and patterns that might be missed by human analysts.
- Sentiment Analysis: Go beyond the words and understand the emotions behind them. AI can detect positive, negative, or neutral sentiment in your data, providing a deeper understanding of stakeholder attitudes.
- Longitudinal Analysis: Track changes in sentiment and perceptions over time. AI helps you identify trends and measure the impact of your program on stakeholders.
Popular Qualitative Data Analysis Techniques:
While AI is revolutionizing the field, traditional qualitative data analysis techniques are still valuable tools. Here are a few key approaches:
- Thematic Analysis: Identify, analyze, and report patterns (themes) within the data. This helps you understand the overarching ideas and experiences emerging from your qualitative research.
- Narrative Analysis: Focus on the stories individuals tell about their experiences with your program. By analyzing the content and structure of these narratives, you can uncover hidden meanings and motivations.
- Content Analysis: Categorize and summarize your qualitative data to identify key concepts and trends. This helps you gain a high-level overview of the main topics and ideas within your data set.
Remember, the best approach often involves a combination of these techniques. By leveraging modern data collection methods and AI-powered analysis, you can unlock deeper insights from your qualitative data, leading to more informed decision-making and ultimately, greater social impact.
Conclusion
Qualitative measurements provide invaluable insights into human experiences, perceptions, and narratives. By employing methods such as interviews, focus groups, and observations, researchers can capture the rich, complex data that quantitative methods might miss. These insights are crucial for understanding the underlying motivations and challenges within various social phenomena. Integrating qualitative data into research enables a deeper, more nuanced analysis, fostering more informed and effective decision-making processes. Ultimately, qualitative measurements enrich our understanding and contribute to more holistic and impactful outcomes.